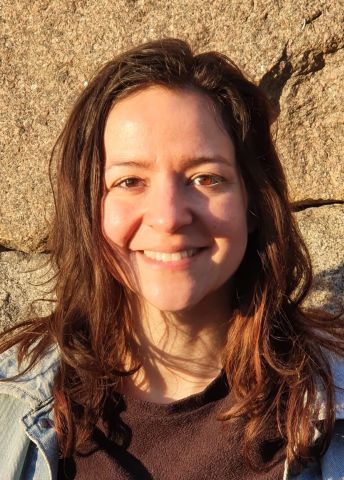
Program for mathematics 2022
Grant to a post-doctoral position abroad
Isabel Haasler
KTH Royal Institute of Technology
Postdoc at the École polytechnique fédérale de Lausanne (EPFL), Switzerland.
Grant to a post-doctoral position abroad
Isabel Haasler
KTH Royal Institute of Technology
Postdoc at the École polytechnique fédérale de Lausanne (EPFL), Switzerland.
Estimating a graph
Isabel Haasler will receive her doctoral degree in mathematics from KTH Royal Institute of Technology in 2022. Thanks to a grant from the Knut and Alice Wallenberg Foundation, she will hold a postdoctoral position with Professor Pascal Frossard at the École polytechnique fédérale de Lausanne (EPFL), Switzerland.
Networks describe many large and complex phenomena, such as biological, financial or societal systems. A network consists of a number of nodes and the edges that connect them. For example, in a social network like Facebook or LinkedIn, each person can be represented by a node, and the contacts between the people represented by edges. Mathematicians call networks graphs, and the purpose of Isabel Haasler’s project is to develop theory for graphs.
Many graphs change over time. For example, users in social networks often make new contacts. So, using knowledge of the graph at several points in time, is it possible to draw conclusions about what it looks like between the known points in time? Is it possible to know what it will look like in the future or what it looked like in the past?
Another question is about systems that consist of multiple graphs, where different types of interactions between the nodes are possible. For example, a social network can be described using several graphs, where the edges of one graph describe which people follow each other, and another graph shows which ones have common interests.
The task is to be able to find a graph that can either describe the network at another point in time or summarise different types of important connections. However, graphs are very special objects that are difficult to compare with each other in a mathematically meaningful manner. New methods that are under development build upon concepts from optimal transport theory and will lead to a flexible and reliable framework for estimating compering graphs that can be used in many different applications.